Enabling the future of CFD for safety applications with Exascale systems
On June 11th, 2019 In Norway, a hydrogen refuelling station exploded. The whole hydrogen refueling station network had to be shut down. Toyota and Hyundai are both halting fuel cell sales in Norway. Elektrek – Hydrogen Station expoldesAs transportation companies struggle to find the way out of fossil fuels, this event illustrates how safety aspects can become a brutal showstopper. In particular, accelerating too much the release to market can jeopardise the efforts of a whole community. Since the public is now aware of the climate urgency, and the competition is open as ever, the industry needs tools to understand the process involved, and test solutions.
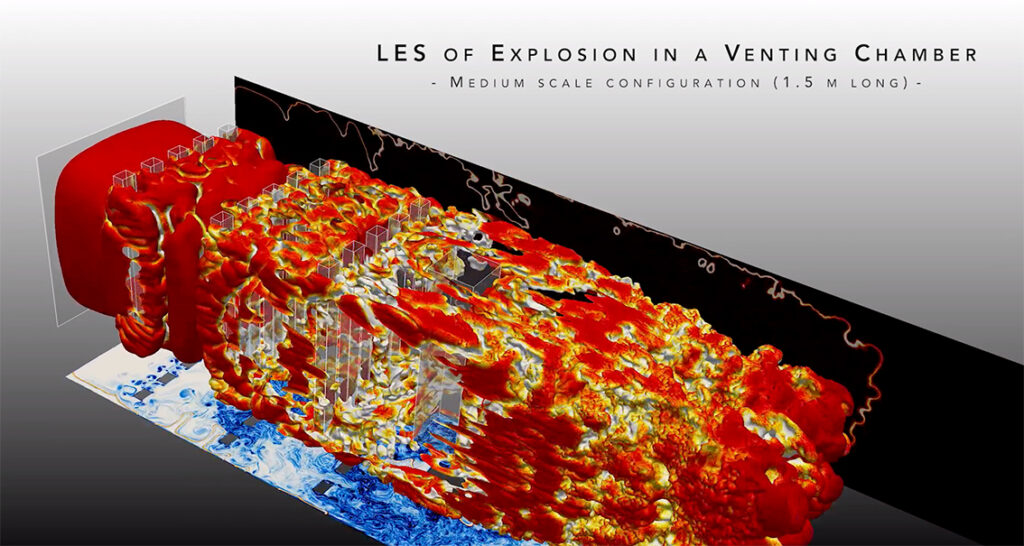
At Cerfacs, we work on such scenarios, for example predicting explosions, but from a numerical point of view.We are especially focused on explosions occurring in industrial Buildings find Video here. The challenge today for these computations is to be able to forecast the combustion process from its initial stage (1cm wide) to the whole building (10m to 100m). A key element in these simulations is the multi-scale aspects with very small flame fronts and the large geometric details of the building. Current state of the art requires fully resolved almost uniform meshes. The simulation is then only possible in medium case (a couple of meters for the domain) and require access to the top10 computers in the world* .
In Excellerat, a European initiative to ensure that the next generation of super computer in Europe will allow to tackle current and future Engineering problems. With this in mind, we are working on an adaptative mesh refinement solution that would reduce mesh and computation requirements by at least one order of magnitude thus allowing current simulation to be performed on more
affordable computer and to tackle more complex cases using the upcoming exascale computers. The first developments are on-going, as of today we are able to use the computed fields to generate and a mesh automatically.
—Gabriel Staffelbach, CERFACS
* Vermorel, O., Quillatre, P. and Poinsot, T. (2017) LES of explosions in venting chamber: a test case for premixed turbulent combustion models, Combustion and Flame, 183 (september), pp. 207-223