Toward Quantifying Uncertainties in Large-Scale Simulations of Engineering Flows
Understanding the complex physics of wall-bounded turbulent flows is of utmost importance, considering the presence of this type of flows in various engineering applications. To this end, the use of high-fidelity approaches such as DNS (Direct Numerical Simulation) and LES (Large Eddy Simulation) has proven to be advantageous. There are, however, at least two main challenges that these approaches have to deal with.
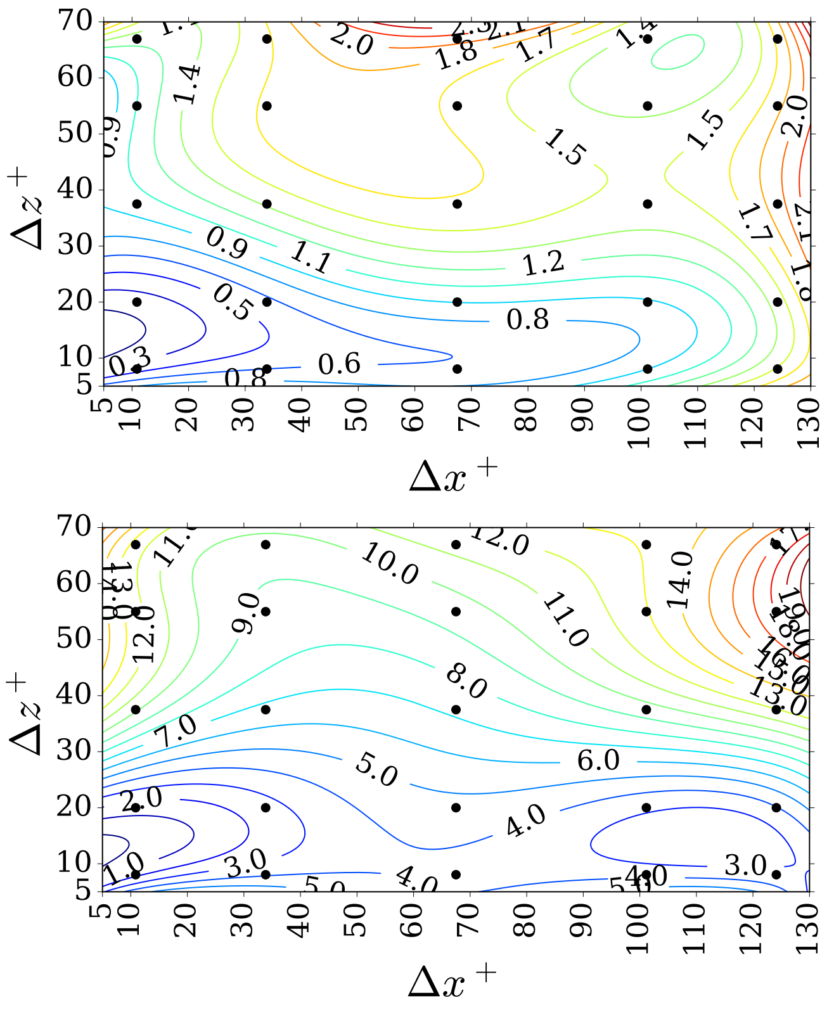
The first is the high computational cost imposed by the requirement of accurately resolving the near-wall region. As a result of the large growth rate of the cost with flow Reynolds (Re) numbers, scale-resolving approaches for many industrial applications are expected to be still out of reach over the future couple of decades, see [1]. Despite this, in moderate range of Re-number, scale-resolving approaches can be successfully employed for complex flows, thanks to the development of the HPC technologies and availability of techniques such as AMR (adaptive mesh refinement) [2] for efficient computing.
In general, the numerical solutions of the Navier-Stokes equations for turbulent flows can be contaminated by some level of uncertainties and errors originating from different sources. These include, but are not limited to, the projection and discretization of the Navier-Stokes
equations, velocity-pressure coupling, imposing initial and boundary conditions, topology and mesh quality, modeling of unresolved structures, bugs in the code, limited sampling time, etc.Hence, the second challenge is to efficiently evaluate how certain and reliable the simulated flow quantities of interest (QoIs) are. Efforts in this direction can be made by the techniques provided within the field of Uncertainty Quantification (UQ). Independent of their fidelity level, the computational codes for exascale HPC are required to be equipped with relevant UQ tools, as clearly advised in NASA’s CFD (Computational Fluid Dynamics) vision 2030 [1].
Our group at KTH Royal Institute of Technology is working on the development and implementation of different UQ techniques in connection with scale-resolving simulations of wall-bounded turbulent flows. Simulations are mainly performed using Nek5000, a high-order scalable open-source CFD code. In order to tackle a wide range of theoretical and engineering problems, the implementation of different UQ techniques is pursued in their most general and flexible form. Of particular interest for us is to investigate how uncertainties from different parameters and inputs propagate to the flow QoIs. For instance, Fig.1 illustrates how variation of the grid resolution in the wall-parallel directions affects the error in the mean velocity and turbulent kinetic energy profiles of a turbulent channel flow. To perform this UQ forward problem, the generalized polynomial chaos expansion (gPCE) technique [3] is used. The pattern of the error isolines depends on the flow solver and the QoI, and it can be rather complex and unexpected, see also [4]. As a complement to the forward problem, global sensitivity analysis which is much more informative than the classical local sensitivity analysis is carried out. As an indication, the Sobol indices [5] in Fig.2 provide a quantitative ground to investigate the sensitivity of the error in different QoIs of a turbulent channel flow with respect to variation of grid resolutions in wall-parallel directions.
In the second major types of problems that are referred to as UQ inverse problems, the goal is to find a combination of Inputs and model parameters which lead to a target value of QoIs (e.g. demanded by an engineering design). Taking into account the complexities and costs involved in numerical simulation of turbulent flows, specific techniques are required to be practiced. The Bayesian inference combined with Gaussian processes can be advantageous in this regard.
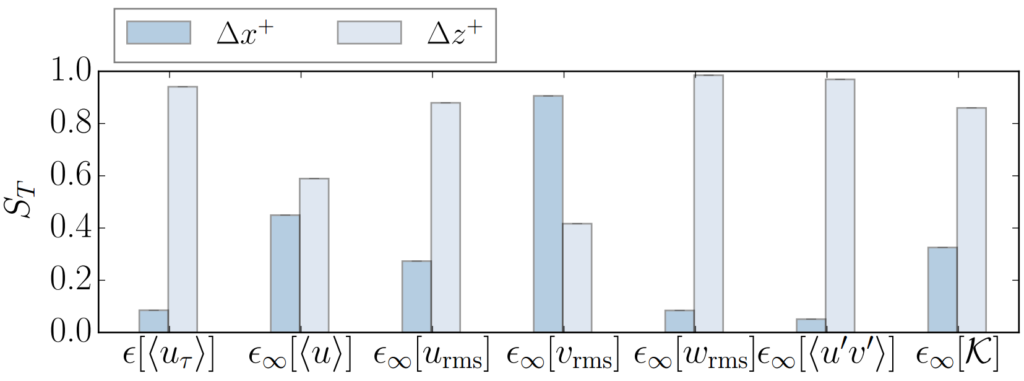
In addition to these, we are attempting to deliver estimators for uncertainties due to the lack of time samples and also being focused on the development of data-driven and multi-fidelity models for simulation of turbulence. These not only lead to more reliable simulations but also can help reduce the overall computational cost to attain a desired level of certainty in the flow QoIs.